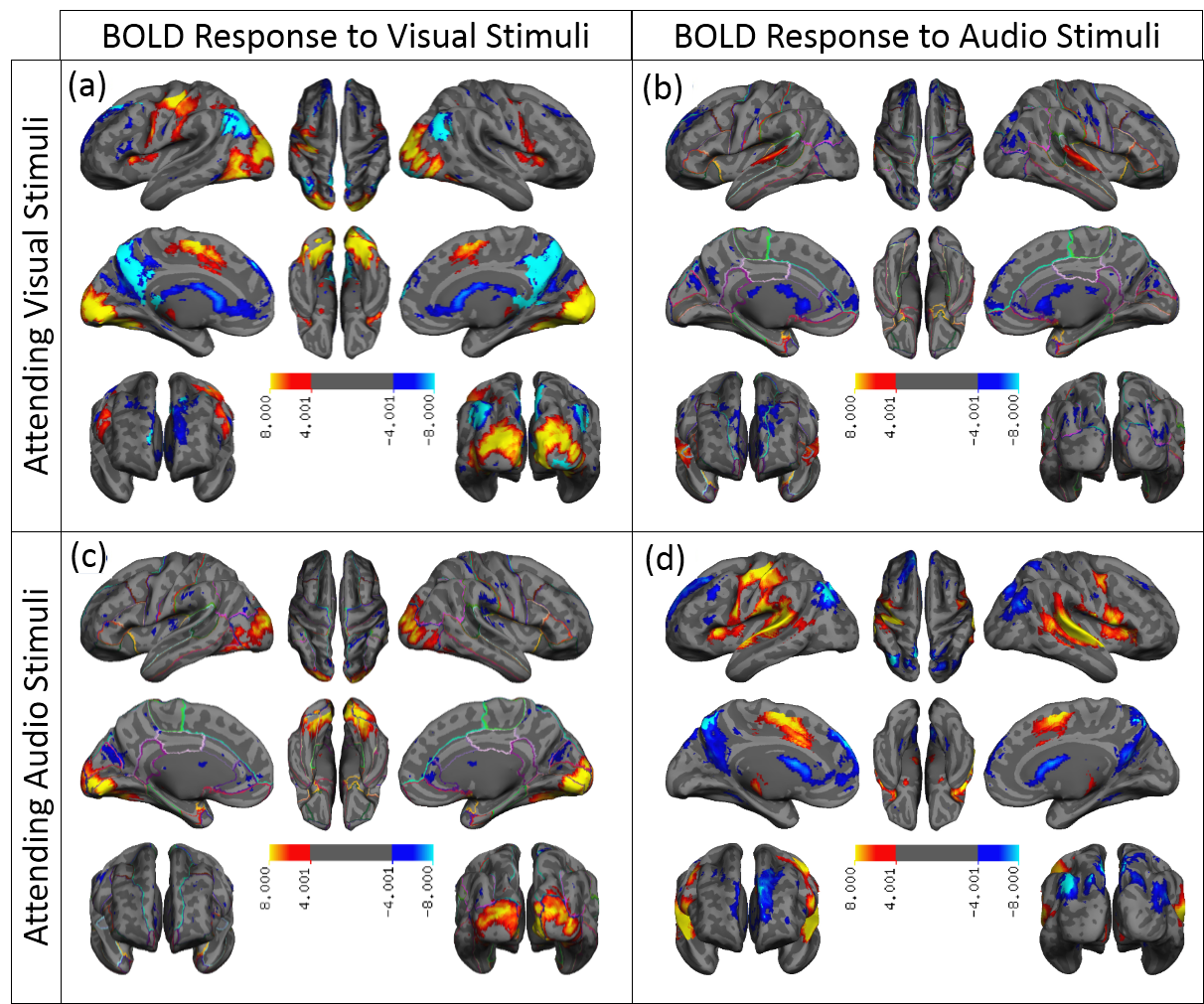
Negative BOLD Response
This project aims to investigate the neural and neurophysiological mechanisms underpinning negative BOLD response in fMRI signal. Although there is emerging evidence that sheds light on the mechanism underlying task-based positive BOLD response, the accompanying negative BOLD response is mainly unknown, even though studies investigating NBR started as early as the introduction of the BOLD-fMRI. One reason for this lack of progress is the possibility that brain regions exhibiting negative BOLD response for a specific task may have separate underlying mechanisms.Therefore they need to be investigated separately.
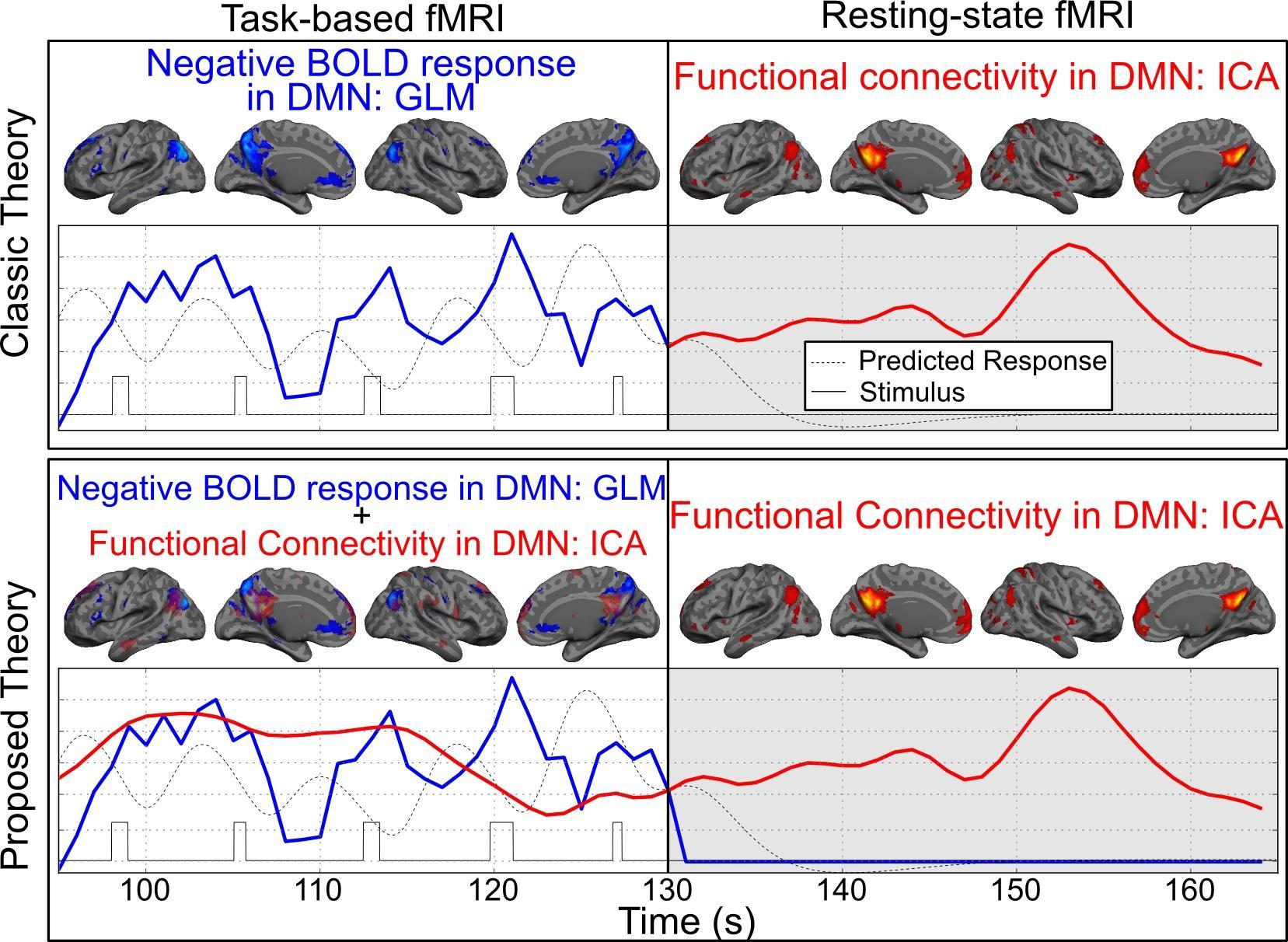
Human Brain's Large-scale Networks
From advances in imaging technology, we have learned that the brain has different networks. Different networks are used during different tasks. They work together to complete any task. One of the most studied brain networks is the default mode network (DMN). It is believed that the DMN is involved in memory and brain activity during rest. Problems with the DMN network occur in normal aging, stages of Alzheimer's disease, and mild cognitive impairment. However, we do not know much about the internal workings of this network. Better understanding how this network works may help us better understand how it fails in diseases such as AD.
Aging and Neurodegenerative Disorders
Amyloid-plaques and Tau-tangles, the well-recognized pathology of Alzheimer’s disease (AD), are often observed in healthy people. Using recent advances in brain imaging technology, we hypothesize and provide evidence that the cognitive symptoms of AD begin only when both pathologies spread to the same brain regions so that they spatially overlap. In contrast, non-overlapping pathologies have only mild effects on brain function to the point that they can be considered a normal part of aging.
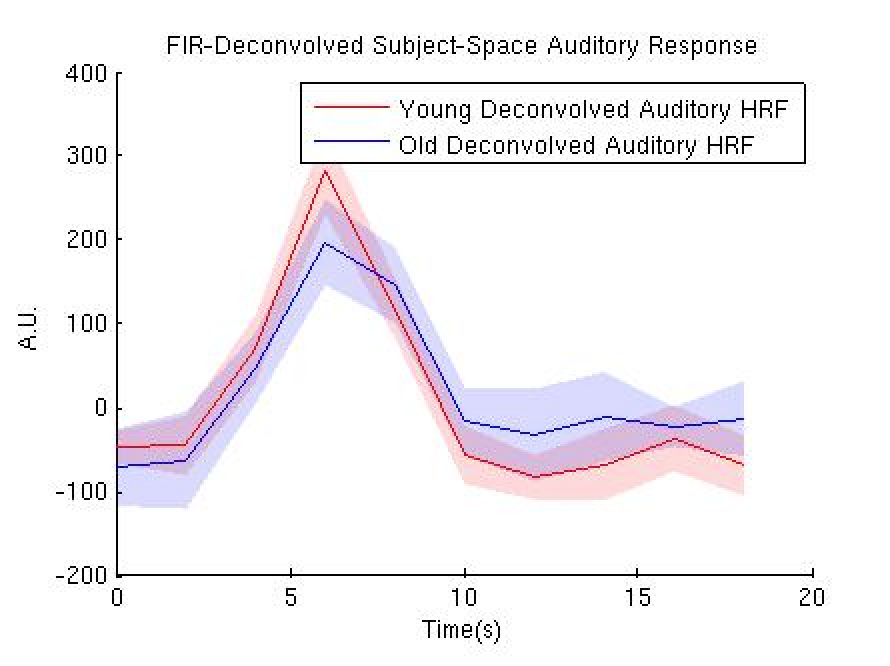
Pre-processing of fMRI data
fMRI data carries BOLD signals, along with many different kind of contaminations, artifacts and noise. Despite the widespread use of the fMRI, there is currently no agreed upon way to optimally extract the underlying BOLD signal. In this project we use basic signal processing techniques to optimally extract BOLD signal.
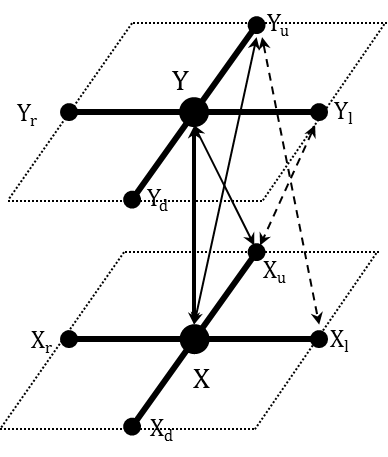
Multilateral Markov Random Field and Brain Subcortical Segmentation
The joint distribution of a causal MRF is readily given by the product of the low-dimensional local distribution, whereas in conventional MRF it is only given through Gibbs distribution. This makes the model closely tied to the image in use. Using MMRF we have developed a Bayesian classifier, called MMRF-MAP, which can be used for segmentation of the subcortical regions.